Revolutionizing AI With the Power of Energy Efficiency
Revolutionizing AI With the Power of Energy Efficiency SciTechDaily
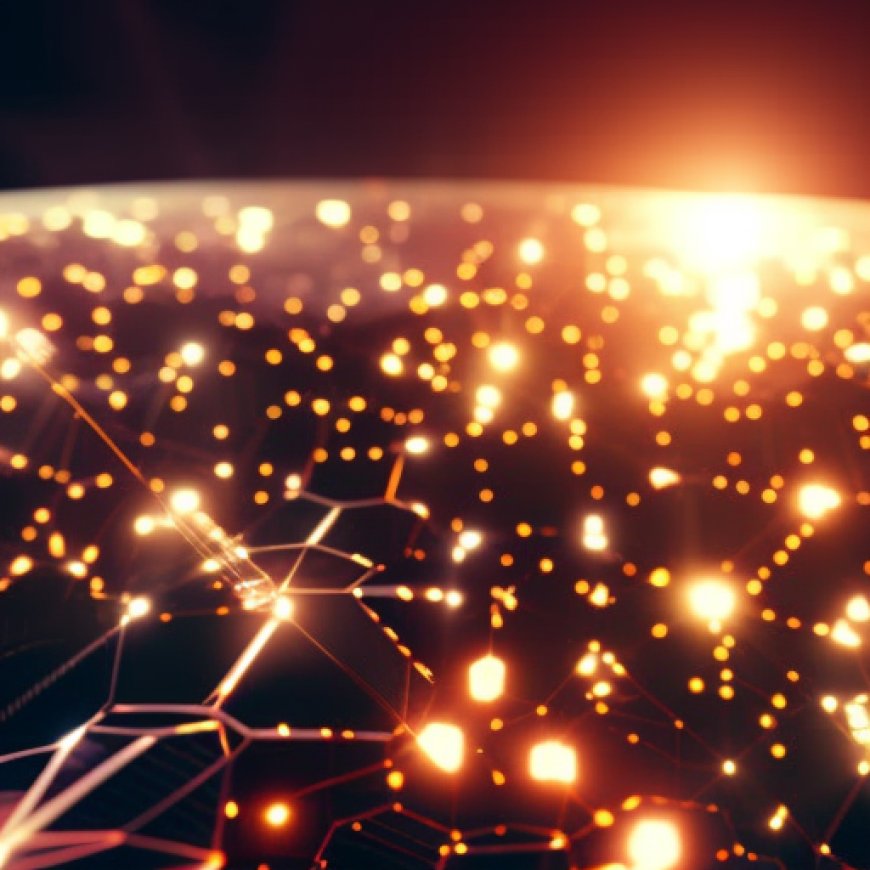
The Development of Energy-Efficient AI Models: A Recipe Book for Sustainability
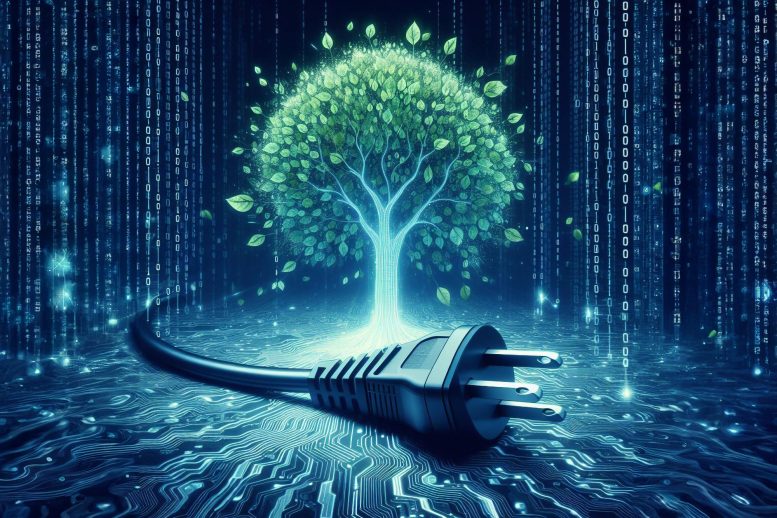
Researchers show how AI’s energy consumption can be greatly reduced with energy-efficient models, leading to substantial energy savings without significantly affecting performance. They provide a guide for AI development prioritizing energy efficiency. Credit: SciTechDaily.com
Introduction
The University of Copenhagen’s computer scientists have recognized the development of AI models as a significant contributor to climate change. In response, they have created a recipe book for designing energy-efficient AI models that maintain high performance. The researchers emphasize the importance of considering a model’s energy consumption and carbon footprint during its design and training phases.
The Impact of AI on Energy Consumption
It is widely known that AI applications, such as Google, Siri, and ChatGPT, consume massive amounts of energy. According to a study, AI servers are projected to consume as much energy as entire countries like Argentina or Sweden by 2027. The energy consumption of a single ChatGPT prompt is estimated to be equivalent to forty mobile phone charges. Despite this knowledge, the research community and industry have yet to prioritize the development of energy-efficient AI models.
The Shift Towards Energy-Efficient AI
Assistant Professor Raghavendra Selvan highlights that current AI model development focuses primarily on accuracy without considering energy consumption. This approach leads to inefficient models in terms of energy usage. Selvan emphasizes the need for energy efficiency to be a fixed criterion in AI model design to reduce carbon footprint.
Why Is AI’s Carbon Footprint So Big?
Training AI models requires extensive energy consumption and results in significant CO2 emissions. The intensive computations involved in model training, especially for large models like ChatGPT’s language model, contribute to high energy usage. Data centers, where AI tasks are processed, demand substantial power for operation and cooling, potentially relying on fossil fuels and increasing their carbon footprint.
Recipe Book for the AI Industry
The researchers conducted calculations to determine the energy required to train over 400,000 convolutional neural network (CNN) AI models. CNNs are commonly used for medical image analysis, language translation, and object and face recognition. Based on their calculations, the researchers present a benchmark collection of AI models that achieve similar performance levels while using significantly less energy. By selecting different models or adjusting existing models, energy savings of 70-80% can be achieved during training and deployment phases, with minimal performance decrease.
Equals 46 Years of a Dane’s Energy Consumption
The study estimates that training the 429,000 neural networks analyzed would require 263,000 kWh of energy. This amount is equivalent to the average energy consumption of a Danish citizen over 46 years. Training these models would take approximately 100 years for a single computer. However, the researchers estimated these energy requirements using another AI model, saving 99% of the energy that would have been consumed.
The researchers describe their findings as a recipe book for AI professionals, providing information on both performance and energy efficiency. By selecting the appropriate model from the beginning, practitioners can significantly reduce energy consumption during training. While precision is crucial in certain fields like self-driving cars and medicine, the researchers emphasize the importance of striving for high energy efficiency in other domains.
The “recipe book” created by the researchers is an open-source dataset available on GitHub for further experimentation by other researchers. It contains information on 423,000 architectures, allowing AI practitioners to access it using simple Python scripts.
Conclusion
To ensure sustainable and responsible AI development, a holistic approach is needed that considers both model performance and climate impact. The University of Copenhagen’s research provides valuable insights into designing energy-efficient AI models without compromising performance. By prioritizing energy efficiency, the AI industry can contribute to achieving the Sustainable Development Goals (SDGs) outlined by the United Nations.
Reference: “EC-NAS: Energy Consumption Aware Tabular Benchmarks for Neural Architecture Search” by Pedram Bakhtiarifard, Christian Igel, and Raghavendra Selvan, 18 March 2024, ICASSP 2024 – 2024 IEEE International Conference on Acoustics, Speech and Signal Processing (ICASSP). DOI: 10.1109/ICASSP48485.2024.10448303
The scientific article about the study will be presented at the International Conference on Acoustics, Speech and Signal Processing (ICASSP-2024).
The authors of the article are Pedram Bakhtiarifard, Christian Igel, and Raghavendra Selvan from the University of Copenhagen’s Department of Computer Science.
SDGs, Targets, and Indicators
1. Which SDGs are addressed or connected to the issues highlighted in the article?
- SDG 7: Affordable and Clean Energy
- SDG 9: Industry, Innovation, and Infrastructure
- SDG 13: Climate Action
2. What specific targets under those SDGs can be identified based on the article’s content?
- SDG 7.3: By 2030, double the global rate of improvement in energy efficiency.
- SDG 9.4: By 2030, upgrade infrastructure and retrofit industries to make them sustainable, with increased resource-use efficiency and greater adoption of clean and environmentally sound technologies and industrial processes.
- SDG 13.2: Integrate climate change measures into national policies, strategies, and planning.
3. Are there any indicators mentioned or implied in the article that can be used to measure progress towards the identified targets?
- Energy consumption of AI models
- Carbon footprint of AI models
- Energy savings achieved during training and deployment phases
- Performance of AI models
Table: SDGs, Targets, and Indicators
SDGs | Targets | Indicators |
---|---|---|
SDG 7: Affordable and Clean Energy | SDG 7.3: By 2030, double the global rate of improvement in energy efficiency. | Energy consumption of AI models |
SDG 9: Industry, Innovation, and Infrastructure | SDG 9.4: By 2030, upgrade infrastructure and retrofit industries to make them sustainable, with increased resource-use efficiency and greater adoption of clean and environmentally sound technologies and industrial processes. | Carbon footprint of AI models |
SDG 9.4: By 2030, upgrade infrastructure and retrofit industries to make them sustainable, with increased resource-use efficiency and greater adoption of clean and environmentally sound technologies and industrial processes. | Energy savings achieved during training and deployment phases | |
SDG 13: Climate Action | SDG 13.2: Integrate climate change measures into national policies, strategies, and planning. | Energy consumption of AI models |
Note: The table above presents the identified SDGs, targets, and indicators based on the analysis of the article.
Behold! This splendid article springs forth from the wellspring of knowledge, shaped by a wondrous proprietary AI technology that delved into a vast ocean of data, illuminating the path towards the Sustainable Development Goals. Remember that all rights are reserved by SDG Investors LLC, empowering us to champion progress together.
Source: scitechdaily.com
Join us, as fellow seekers of change, on a transformative journey at https://sdgtalks.ai/welcome, where you can become a member and actively contribute to shaping a brighter future.