Associations of long-term exposure to air pollution and physical activity with the risk of systemic inflammation-induced multimorbidity in Chinese adults: results from the China multi-ethnic cohort study (CMEC) – BMC Public Health
Associations of long-term exposure to air pollution and physical activity with the risk of systemic inflammation-induced ... BMC Public Health
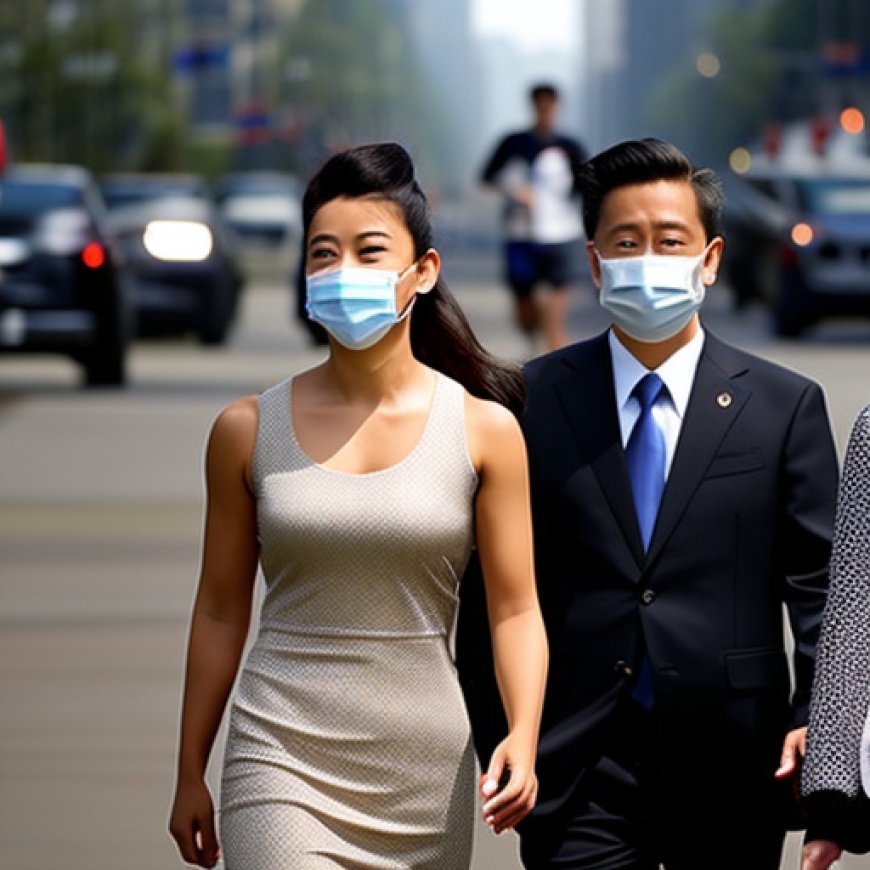
Study Population
The study populations were derived from the China Multi-Ethnic Cohort study (CMEC). A total of 99,556 participants aged 30–79 years old in Southwestern China were recruited from May 2018 to September 2019 by multistage, stratified cluster sampling method. Data were collected by questionnaires, medical examinations, and clinical laboratory tests. Through face-to-face interviews via electronic questionnaires, the CMEC study elicited information on demographic, socioeconomic, health behaviors, family disease history, physician-diagnosed diseases, indoor air pollution, and other health-related factors. A range of medical examinations, including physical examination, chest radiography, osteopathic examination, and abdominal ultrasonography, were performed to diagnose some physical diseases (e.g., hypertension). In addition, venous blood samples were collected after overnight fasting (at least 8 hours) for clinical laboratory tests, that is, blood routine, fasting blood glucose, blood lipid levels, and liver function. More details on the CMEC study have been reported previously [25]. This study was approved by Sichuan University Medical Ethical Review Board (K2016038, K2020022). Written consents from all participants were obtained.
Exposures Assessment
A detailed description of the exposure assessment was described elsewhere [26]. In brief, daily concentrations of 3 air pollutants (PM1, PM2.5, and PM10) were predicted by the space-time extremely randomized trees model using aerosol optical depth, land use information, topographical, and meteorological data [27, 28]. Three-year average concentrations of each air pollutant of participants before the baseline survey were calculated and developed as substitutes for long-term air pollution exposure according to geocoded residential addresses.
Outcome Assessment
Systemic inflammation-induced multimorbidity (SIIM) including 11 CIDs among the Chinese population [31], was treated as the outcome of our studies. Among these CIDs, definitions of hypertension, diabetes, and metabolic associated fatty liver disease (MAFLD) were based on questionnaires or medical examinations. Seven diagnosed chronic diseases were self-reported (pulmonary heart disease, rheumatic heart disease, coronary heart disease, rheumatic arthritis, stroke, cancer, and rheumatoid arthritis); chronic kidney disease (CKD) was measured by medical examinations. SIIM was defined as having at least two of the defined CIDs [1, 4].
Covariates
Based on the previous literature on air pollution and chronic diseases [37], fully adjusted models included the following other covariates through questionnaires and medical examinations: age (continuous), sex (male or female), marital (cohabited and did not cohabit), ethnic group (Han and minority), region (Yunnan, Guizhou, Chongqing, and Sichuan), annual family income (< 20,000 yuan, 20,000–59,999 yuan, or ≥60,000 yuan), educational level (illiteracy, primary school, junior high school, and high school or above), smoking (never, former, and current), secondary smoking (yes and no), alcohol drinking (never, occasionally, and often), sleep duration (< 6 hours per night, 6–8 hours per night, and > 8 hours per night), indoor air pollution (low, moderate, and high level), body mass index (BMI) (continuous), and dietary pattern (continuous). Indoor air pollution was divided into low, moderate, or high levels according to a summary of cooking behavior, fuel types, and ventilation equipment [26]. BMI was calculated as the body weight (kg) divided by the height squared (m^2). The dietary pattern was evaluated by the Dietary Approaches to Stop Hypertension (DASH) diet score, which emphasized the consumption of fruits, vegetables, nuts, sodium, low-fat dairy, red and processed meats, and whole-grain intake [38,39,40].
Statistical Analysis
Differences in essential characteristics between participants with and without SIIM were presented as mean ± standard deviation (SD) and numbers (percentages), and were tested using Student’s t-test, the Wilcoxon rank-sum test, and the chi-square test. Pearson correlation test was used to assess the correlation between air pollutants. Logistic regression models were performed to investigate associations of air pollutants (per 10 µg/m^3 increase) with the risk of SIIM after adjusting potential confounders. Model 1 was adjusted for age, sex, region, ethnic group, marital, annual family income, educational level, smoking, secondary smoking, alcohol drinking, sleep duration, BMI, dietary pattern, and indoor air pollution. Model 2 additionally adjusted for PA and was the main model because it accounted for the most comprehensive covariates. These results are presented as odds ratios (ORs) with 95% confidence intervals (CIs). Given the high correlations among air pollutants, only single-pollutant models were applied.
Stratified Analysis
We conducted a stratified analysis to investigate associations of PA with SIIM in different levels of air pollution subgroups. In this analysis, the reference group was set as the participants engaged in a high volume of PA based on their tri-sectional quantile range of metabolic equivalent tasks per day, and we examined whether low PA was associated with SIIM across different air pollution subgroups.
Interaction Analysis
To quantify the additive and multiplicative interactions, we additionally included a product term of air pollution and PA in the model. The odds ratios (ORs) with 95% confidence intervals (CIs) of the product term were the measure of interaction on the multiplicative scale. We used the relative excess risk due to interaction (RERI) and corresponding 95% confidence intervals (CIs) as the measure of interaction on the additive scale, calculated using the coefficients and corresponding standard errors of the product term, air pollution, and PA, as well as covariance matrix [41].
Sensitivity Analysis
All of the analyses were performed using R 4.0.2 (R Foundation for Statistical Computing), with a P-value < 0.05 considered statistically significant for a two-tailed test.
SDGs, Targets, and Indicators
SDGs Addressed or Connected to the Issues Highlighted in the Article:
- SDG 3: Good Health and Well-being
- SDG 11: Sustainable Cities and Communities
- SDG 13: Climate Action
Specific Targets Under Those SDGs Based on the Article’s Content:
- SDG 3.4: By 2030, reduce by one third premature mortality from non-communicable diseases through prevention and treatment and promote mental health and well-being.
- SDG 11.6: By 2030, reduce the adverse per capita environmental impact of cities, including by paying special attention to air quality and municipal and other waste management.
- SDG 13.1: Strengthen resilience and adaptive capacity to climate-related hazards and natural disasters in all countries.
Indicators Mentioned or Implied in the Article:
- Air pollutant concentrations (PM1, PM2.5, PM10) as indicators of environmental impact (related to SDG 11.6 and SDG 13.1).
- Prevalence of systemic inflammation-induced multimorbidity (SIIM) as an indicator of premature mortality from non-communicable diseases (related to SDG 3.4).
The article discusses the health effects of air pollution on the Chinese population, specifically focusing on the association between air pollutants (PM1, PM2.5, PM10) and the risk of systemic inflammation-induced multimorbidity (SIIM), which includes various chronic diseases. This issue is directly connected to SDG 3 (Good Health and Well-being) as it aims to reduce premature mortality from non-communicable diseases. The article also highlights the importance of addressing air pollution in cities, which is relevant to SDG 11 (Sustainable Cities and Communities) and its target to reduce the adverse environmental impact of cities, including air quality. Additionally, the article mentions the need to strengthen resilience to climate-related hazards, which aligns with SDG 13 (Climate Action) and its target to enhance adaptive capacity to climate change.
Based on the content of the article, the specific targets that can be identified include SDG 3.4 (reduce premature mortality from non-communicable diseases), SDG 11.6 (reduce adverse environmental impact of cities), and SDG 13.1 (strengthen resilience to climate-related hazards). These targets reflect the goals of improving health outcomes, promoting sustainable urban development, and addressing climate change impacts.
The indicators mentioned or implied in the article include air pollutant concentrations (PM1, PM2.5, PM10) as indicators of environmental impact, specifically related to air quality and municipal waste management in cities (SDG 11.6). The prevalence of systemic inflammation-induced multimorbidity (SIIM) is also mentioned as an indicator of premature mortality from non-communicable diseases (SDG 3.4). These indicators provide measurable data to assess progress towards the identified targets.
Table: SDGs, Targets, and Indicators
SDGs | Targets | Indicators |
---|---|---|
SDG 3: Good Health and Well-being | Target 3.4: By 2030, reduce by one third premature mortality from non-communicable diseases through prevention and treatment and promote mental health and well-being. | Prevalence of systemic inflammation-induced multimorbidity (SIIM) |
SDG 11: Sustainable Cities and Communities | Target 11.6: By 2030, reduce the adverse per capita environmental impact of cities, including by paying special attention to air quality and municipal and other waste management. | Air pollutant concentrations (PM1, PM2.5, PM10) |
Target 11.6: By 2030, reduce the adverse per capita environmental impact of cities, including by paying special attention to air quality and municipal and other waste management. | Air pollutant concentrations (PM1, PM2.5, PM10) | |
SDG 13: Climate Action | Target 13.1: Strengthen resilience and adaptive capacity to climate-related hazards and natural disasters in all countries. | Air pollutant concentrations (PM1, PM2.5, PM10) |
Behold! This splendid article springs forth from the wellspring of knowledge, shaped by a wondrous proprietary AI technology that delved into a vast ocean of data, illuminating the path towards the Sustainable Development Goals. Remember that all rights are reserved by SDG Investors LLC, empowering us to champion progress together.
Source: bmcpublichealth.biomedcentral.com
Join us, as fellow seekers of change, on a transformative journey at https://sdgtalks.ai/welcome, where you can become a member and actively contribute to shaping a brighter future.