Planet Data Leveraged to Understand Agricultural Land and Qu…
Planet Data Leveraged to Understand Agricultural Land and Qu... planet.com
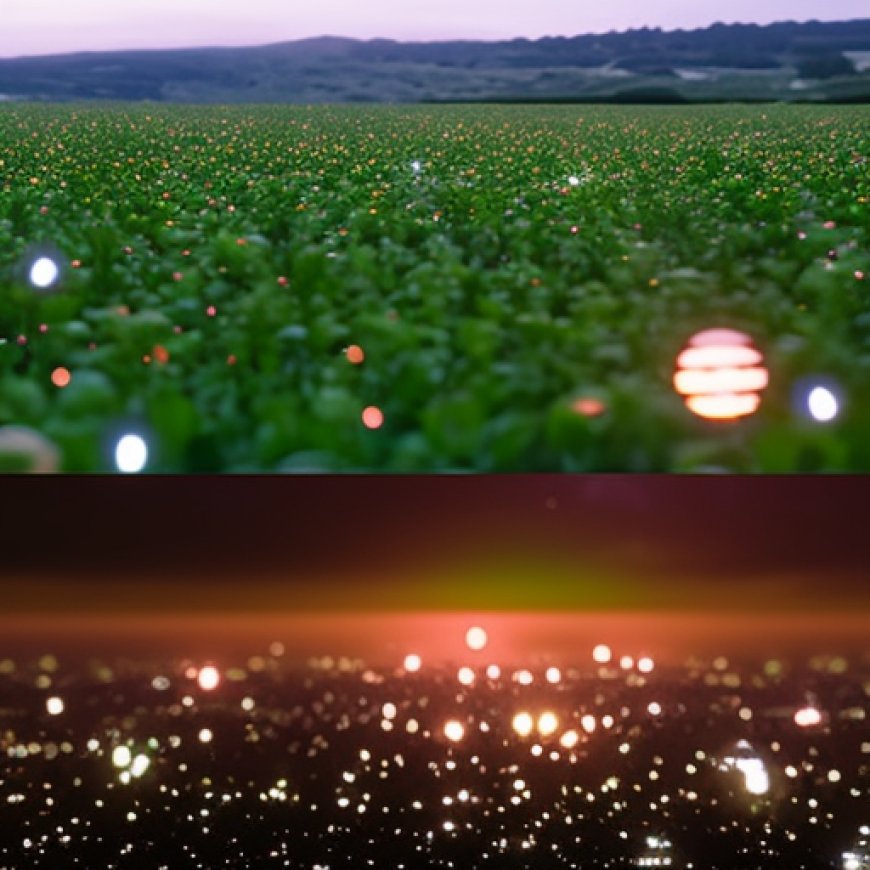
We are excited to share the power of Planet satellite imagery with Amazon SageMaker’s geospatial capabilities
We are excited to announce our partnership with Amazon SageMaker, which combines Planet satellite imagery with machine learning and cloud architecture to revolutionize agriculture and sustainability insights. By leveraging geospatial data and advanced technologies, we can drive informed decisions, enhance climate resilience, and enable rapid response in various applications.
Informed Decisions
- By gaining better spatial understanding of croplands, farmers can optimize resource allocation, fostering sustainable practices.
Climate Resilience
- Satellite imagery aids in identifying climate-stressed regions, facilitating adaptive strategies to combat climate change impacts.
Rapid Response
- Swift crop segmentation assists in quickly identifying post-disaster damaged areas, streamlining relief efforts.
Amazon SageMaker’s geospatial capabilities empower data scientists and machine learning engineers to construct, train, and deploy models using geospatial data. The partnership between Planet and Amazon SageMaker provides users access to Planet satellite data via SageMaker, enabling a seamless integration and faster results.
Build a crop segmentation machine learning model with Planet data and Amazon SageMaker geospatial capabilities
This guest post is co-written by Lydia Lihui Zhang, Business Development Specialist, and Mansi Shah, Software Engineer/Data Scientist, at Planet Labs. The analysis that inspired this post was originally written by Jennifer Reiber Kyle.
Amazon SageMaker geospatial capabilities combined with Planet satellite data can be used for crop segmentation, offering numerous applications and potential benefits in agriculture and sustainability. In late 2023, Planet announced a partnership with AWS to make its geospatial data available through Amazon SageMaker.
Crop segmentation is the process of splitting up a satellite image into regions of pixels that have similar crop characteristics. By using a segmentation machine learning (ML) model, we can identify crop and non-crop regions in an image.
Identifying crop regions is crucial for gaining agricultural insights and driving data-driven farming decisions. It also helps in identifying climate-related stresses and trends, enabling climate adaptation strategies. Additionally, crop segmentation can assist in assessing and mitigating damage by quickly identifying post-disaster damaged areas.
With Amazon SageMaker geospatial capabilities, data scientists and ML engineers can efficiently transform or enrich large-scale geospatial datasets, accelerate model building with pre-trained ML models, and explore model predictions and geospatial data on an interactive map. Planet Labs’ high-resolution satellite imagery provides valuable geospatial data for training and testing ML models.
Data Selection
The agricultural field selected for this analysis is located in Sacramento County, California. The availability of ground truth data from the 2015 Sacramento County Land Use DWR Survey dataset makes it an ideal choice for this study. Planet’s 4-band PSScene Product, which contains Blue, Green, Red, and Near-IR bands, is used as the primary satellite imagery.
Access Planet Data
Planet has developed the Planet Software Development Kit (SDK) for Python, which allows users to search and access Planet’s collection of high-resolution satellite imagery. The SDK provides a Python client to Planet’s APIs, making it easy to incorporate satellite imagery and geospatial data into Python workflows.
Model Training
The trained K-nearest neighbors (KNN) classifier is used to predict crop regions in the test dataset. The classifier’s performance is evaluated using classification metrics such as precision, recall, and F1-score. The results demonstrate the effectiveness of the KNN classifier in accurately identifying crop regions in remote sensing images.
Create a Segmentation Mask
A segmentation mask is created using the predictions from the KNN classifier on the test dataset. The predicted output is cleaned up to remove small segments caused by image noise. The denoised output is then converted into vector features and used to compute the segmented crop regions.
Conclusion
The combination of Planet’s satellite imagery and Amazon SageMaker’s geospatial capabilities offers valuable insights for improving agricultural efficiency, environmental sustainability, and food security. Accurately identifying crop regions enables further analysis on crop growth and productivity, monitoring of land use changes, and detection of potential food security risks.
The partnership between Planet and Amazon SageMaker provides users with access to high-frequency satellite data and advanced machine learning tools, enabling diverse applications in various industries. By leveraging geospatial data and ML models, we can drive digital transformation, sustainability transformation, and security.
To start using Amazon SageMaker geospatial capabilities, visit the AWS website. For more information about Planet’s imagery specifications and developer reference materials, visit the Planet Developer’s Center.
Planet Labs PBC Forward-Looking Statements
Except for the historical information contained herein, the matters set forth in this blog post are forward-looking statements within the meaning of the “safe harbor” provisions of the Private Securities Litigation Reform Act of 1995. Actual results may differ materially from those projected due to various factors. For more information, please refer to Planet Labs PBC’s periodic reports filed with the Securities and Exchange Commission (SEC).
SDGs, Targets, and Indicators
SDG 2: Zero Hunger
- Target 2.4: By 2030, ensure sustainable food production systems and implement resilient agricultural practices that increase productivity and production, that help maintain ecosystems, that strengthen capacity for adaptation to climate change, extreme weather, drought, flooding and other disasters, and that progressively improve land and soil quality.
- Indicator 2.4.1: Proportion of agricultural area under productive and sustainable agriculture.
SDG 13: Climate Action
- Target 13.1: Strengthen resilience and adaptive capacity to climate-related hazards and natural disasters in all countries.
- Indicator 13.1.1: Number of deaths, missing persons and directly affected persons attributed to disasters per 100,000 population.
SDG 15: Life on Land
- Target 15.3: By 2030, combat desertification, restore degraded land and soil, including land affected by desertification, drought and floods, and strive to achieve a land degradation-neutral world.
- Indicator 15.3.1: Proportion of land that is degraded over total land area.
SDG 17: Partnerships for the Goals
- Target 17.6: Enhance North-South, South-South and triangular regional and international cooperation on and access to science, technology and innovation and enhance knowledge sharing on mutually agreed terms, including through improved coordination among existing mechanisms, in particular at the United Nations level, and through a global technology facilitation mechanism.
- Indicator 17.6.1: Number of science and/or technology cooperation agreements and programmes between countries, by type of cooperation.
Behold! This splendid article springs forth from the wellspring of knowledge, shaped by a wondrous proprietary AI technology that delved into a vast ocean of data, illuminating the path towards the Sustainable Development Goals. Remember that all rights are reserved by SDG Investors LLC, empowering us to champion progress together.
Source: planet.com
Join us, as fellow seekers of change, on a transformative journey at https://sdgtalks.ai/welcome, where you can become a member and actively contribute to shaping a brighter future.