Published at Nature – Automatic heliostat learning for in situ concentrating solar power plant metrology with differentiable ray tracing
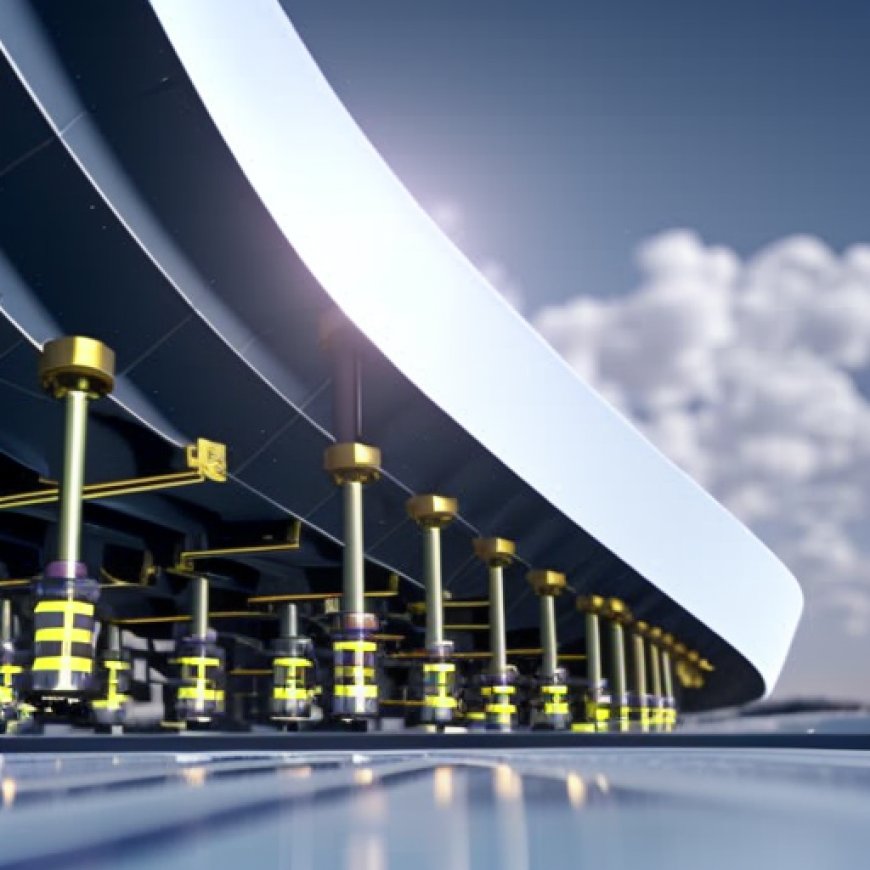
Concentrating Solar Power Plants: A Sustainable Solution for Clean Energy
Abstract
Concentrating solar power (CSP) plants play a vital role in achieving the Sustainable Development Goals (SDGs) by providing a clean energy source for competitive electricity generation, even during night time. These plants also have the potential to produce carbon-neutral fuels, making them a valuable complement to photovoltaic plants. In CSP plants, thousands of mirrors, known as heliostats, redirect sunlight onto a receiver, which can generate temperatures exceeding 1000°C. However, due to operational challenges such as misalignment in sun-tracking and surface deformations, achieving such high temperatures is often difficult. These challenges necessitate the implementation of high safety margins to prevent dangerous temperature spikes. In order to achieve a competitive levelized cost of energy and enable large-scale deployment of CSP plants, it is essential to accurately measure and address these operational errors. Unfortunately, in-situ error measurements have not yet been achieved.
To address this issue, a team of researchers has introduced a novel approach that combines differentiable ray tracing with machine learning. This approach allows for the derivation of the irradiance distribution of heliostats in a data-driven manner using a small number of calibration images, which are already collected in most solar towers. By utilizing gradient-based optimization and a learning non-uniform rational B-spline heliostat model, this approach can accurately determine sub-millimeter imperfections in real-world settings and predict heliostat-specific irradiance profiles. The precision of this approach surpasses that of the current state-of-the-art methods and establishes full automatization. This optimization pipeline also enables concurrent training of physical and data-driven models, representing a pioneering effort in unifying both paradigms for concentrating solar power plants. The findings of this study can serve as a blueprint for other domains seeking to integrate physical and data-driven models.
Reference
- Pargmann, M., Ebert, J., Götz, M., Maldonado Quinto, D., & Kesselheim, S. (2024). Automatic heliostat learning for in situ concentrating solar power plant metrology with differentiable ray tracing. Nature Communications, 15(1), 1-12. https://doi.org/10.1038/s41467-024-51019-z
SDGs, Targets, and Indicators
1. SDGs Addressed or Connected to the Issues Highlighted in the Article:
- SDG 7: Affordable and Clean Energy
- SDG 9: Industry, Innovation, and Infrastructure
- SDG 13: Climate Action
2. Specific Targets Based on the Article’s Content:
- SDG 7.2: Increase substantially the share of renewable energy in the global energy mix.
- SDG 9.4: Upgrade infrastructure and retrofit industries to make them sustainable.
- SDG 13.2: Integrate climate change measures into national policies, strategies, and planning.
3. Indicators Mentioned or Implied in the Article:
- Efficiency of concentrating solar power plants in generating electricity during night time and producing carbon-neutral fuels.
- Measurement of misalignment in sun-tracking and surface deformations to ensure safe operation and prevent temperature spikes.
- Levelized cost of energy and large-scale deployment as indicators of competitiveness and feasibility.
- Derivation of irradiance distribution of heliostats in a data-driven manner.
- Prediction of heliostat-specific irradiance profiles with high precision.
SDGs, Targets, and Indicators Table:
SDGs | Targets | Indicators |
---|---|---|
SDG 7: Affordable and Clean Energy | 7.2: Increase substantially the share of renewable energy in the global energy mix. | – Efficiency of concentrating solar power plants in generating electricity during night time and producing carbon-neutral fuels. |
SDG 9: Industry, Innovation, and Infrastructure | 9.4: Upgrade infrastructure and retrofit industries to make them sustainable. | – Measurement of misalignment in sun-tracking and surface deformations to ensure safe operation and prevent temperature spikes. – Levelized cost of energy and large-scale deployment as indicators of competitiveness and feasibility. |
SDG 13: Climate Action | 13.2: Integrate climate change measures into national policies, strategies, and planning. | – Derivation of irradiance distribution of heliostats in a data-driven manner. – Prediction of heliostat-specific irradiance profiles with high precision. |
Note: The indicators listed in the table are based on the information provided in the article and may not represent an exhaustive list of all possible indicators for each target.
Source: solarpaces.org