Using geospatial data & machine learning to predict water quality in Ethiopia
Using geospatial data & machine learning to predict water quality in Ethiopia World Bank
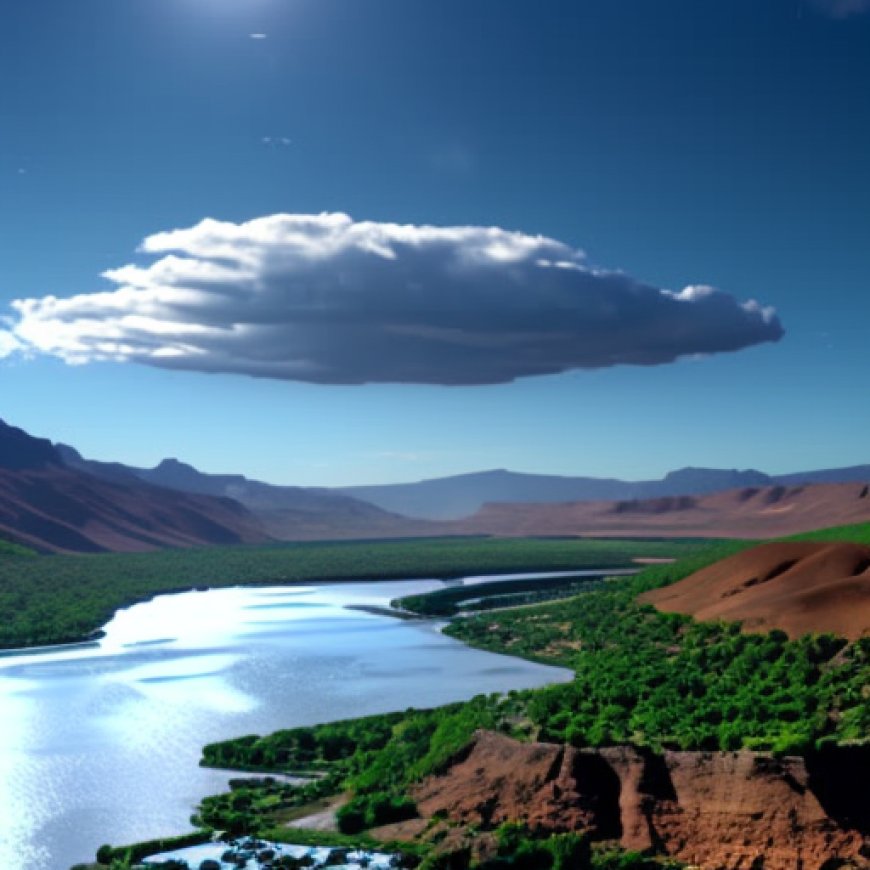
Expanding Access to Safe Drinking Water: A Report on Water Quality Testing

Introduction
Access to safe drinking water is a crucial aspect of human development, and it is a priority at both national and global levels. The Sustainable Development Goals (SDGs) also emphasize the importance of ensuring access to safe water, sanitation, and hygiene for all.
Monitoring Drinking Water Quality
To track progress towards achieving global and national targets, it is essential to have accurate information on drinking water quality. This includes measuring the presence of biological and chemical contaminants in drinking water accessed by households and individuals.
Integration of Water Quality Testing in Household Surveys
Over 50 countries have integrated water quality testing into national household surveys to monitor access to safely managed drinking water services. This approach allows for the collection of representative data for different geographic and socioeconomic groups.
Linking water quality information with other data collected in household surveys enables research and the identification of effective interventions to improve access to safe drinking water services.
However, integrating water quality testing in household surveys requires additional financial resources and specialized technical assistance. It can also increase the burden on statistical agencies, particularly in resource-constrained contexts.
Filling Data Gaps with Data Integration and Machine Learning
In a recent study conducted by the World Bank Living Standards Measurement Study (LSMS) team and the Joint Monitoring Programme (JMP) of the World Health Organization and UNICEF, an approach was proposed to fill data gaps in drinking water quality using data integration and machine learning.
The methodology involved integrating data from recent surveys with publicly available geospatial data on factors such as rainfall, temperature, and proximity to markets and roads. This integrated data was then used to train a machine learning model to generate insights on drinking water quality in years when no surveys were conducted.
Case Study: Ethiopia
The study focused on Ethiopia, where data on water quality was collected as part of the Ethiopian Socioeconomic Survey in 2016. The findings revealed that over half of the improved water sources in the country were contaminated.
Using data from the survey, the study developed a predictive model for E. coli contamination in drinking water sources. Different machine learning algorithms were compared, and the Random Forest algorithm performed the best.
The study also examined the impact of different predictor variables on the performance of the predictive models. It was found that a combination of household demographic and socioeconomic attributes, water service particularities, and geospatial variables resulted in the most accurate predictions.
Key Findings
-
Machine learning approaches can fill gaps in data on drinking water quality when implementing water quality testing is challenging.
-
A georeferenced household survey with objective water testing, integrated with geospatial data sources, can generate reliable predictive models for drinking water quality.
-
Predictive machine learning models relying exclusively on geospatial variables can provide insights into variations in the risk of E. coli contamination and generate water quality risk maps.
Conclusion
The study demonstrates the potential of data integration and machine learning in addressing gaps in data on drinking water quality. By leveraging existing data and geospatial information, reliable insights can be generated to inform interventions and improve access to safe drinking water. To learn more about the study, read the full working paper “Addressing gaps in data on drinking water quality through data integration and machine learning: evidence from Ethiopia” and visit the World Bank’s Living Standards Measurement Study (LSMS) website.
Join us, as fellow seekers of change, on a transformative journey at https://sdgtalks.ai/welcome, where you can become a member and actively contribute to shaping a brighter future.